Evolution of viruses and bacteria
We want to understand the dynamics of evolution and the forces that drive it. Evolution of plants and animals tend to happen over centuries of even longer time-scales. Microbial organisms, in contrast, often adapt to changing environments within weeks. We study such rapidly evolving systems to address fundamental questions in evolutionary biology. Among the most prominent rapidly adapting systems are pathogenic RNA viruses and the study of their evolution can help treatment or outbreak response as well as uncover fundamental mechanisms of evolution.
Real-time tracking and prediction of pathogenic RNA viruses
Seasonal influenza viruses cause 10s to 100s of millions of infections every year. While humans develop lasting immunity against particular influenza virus strains, the viruses change rapidly to evade preexisting immunity and reinfect the same individual multiple times. Similarly, the vaccine against seasonal influenza requires frequent updates to match the circulating viruses. Among the four seasonal influenza virus lineages, the type A/H3N2 changes its antigenic properties most rapidly. The HA segment alone changes at 5-10 of its 1700 positions every year.
To monitor the evolution and spread of seasonal influenza viruses, our group – together with the lab of Trevor Bedford – developed an automated analysis pipeline coupled to an interactive visualization available at the web page nextflu.org. This site is updated at least weakly with the latest available influenza virus sequence data such that public health authorities, scientists, as well as the interested public have access to the latest analysis. We have recently expanded these efforts to other viral pathogens including Ebola virus, Zika virus, Mumps, etc. These analysis are available at nextstrain.org.
The decision on the composition of the influenza vaccine needs to be taken close to year before the vaccine is rolled out. However, influenza population can change considerably over a year and methods to reliably predict future influenza population could potentially improve the match between vaccine and the circulating virus. Together with Boris Shraiman and Colin Russell, we have developed methods to predict fitness and hence future success from a single sample of genomes. This idea emerged from our theoretical study of abstract models of evolution but has immediate applications as a tool to predict influenza virus evolution. We have evaluated this method retrospectively on the influenza seasons 1995-2013 and have since been using it to predict composition of the upcoming influenza seasons. To enable rapid, near real-time phylodynamic analysis of large numbers of viral genomes, we have developed the software suite TreeTime. TreeTime implements an approximate maximum likelihood inference of time-stamped phylogenies and demographic models.
Intra-host dynamics and deep sequencing of HIV
Understanding the evolution of the virus is central to developing vaccines and to minimize drug resistance. In addition, HIV provides an ideal model system in which fundamental questions of evolution can be addressed. In contrast to most other model systems of evolution, time series data is available for HIV evolution which is much more informative about the evolutionary process than the snap shot typical of less rapidly evolving populations.
We performed whole-genome deep sequencing of HIV-1 populations in 9 untreated patients, with 6-12 longitudinal samples per patient spanning 5-8 years of infection. This work was led by Fabio Zanini (http://orcid.org/0000-0001-7097-8539) and is the product of a fruitful collaboration with the group of Jan Albert at the Karolinska Institute in Stockholm. (http://ki.se/en/mtc/jan-albert-group) We show that one third of common mutations are reversions towards the ancestral HIV-1 sequence which occur throughout infection with a rate that increases with conservation. Frequent recombination limits linkage disequilibrium to about 100bp. However, hitch-hiking due to the remaining short range linkage causes levels of synonymous diversity to be inversely related to the speed of evolution (Zanini et al. 2015). All data we generated as part of this project are available at (http://hiv.biozentrum.unibas.ch). We have used these data to estimate the fitness costs at every position of the HIV genome and infer the in vivo mutation rates of the virus (Zanini et al. 2017).
HIV not only evolves fast, but also integrates into the genome of human T-cells as latent provirus. Latent virus is the principal barrier to cure because latent virus reseeds an active HIV infection is therapy is stopped. It is therefore critical to understand how this latent reservoir is maintained. In particular, whether the virus constantly replicates in parts of the body with insufficient drug penetration or whether the virus survives in long-lived T-cell lineages is hotly debated.
We studied the evolution of HIV before and after therapy in great detail and find no evidence of consistent viral evolution after the start of therapy (http://dx.doi.org/10.7554/eLife.18889). To the contrary, virus isolated from the genome of human cells is an almost perfect snapshot of the virus that circulated right before therapy was initiated. The graph below shows that evolutionary distance the virus population diverged over time before and after therapy. Once therapy is started, there is no evidence of further evolution. Latently integrated HIV remains very similar to virus circulating before start of treatment.
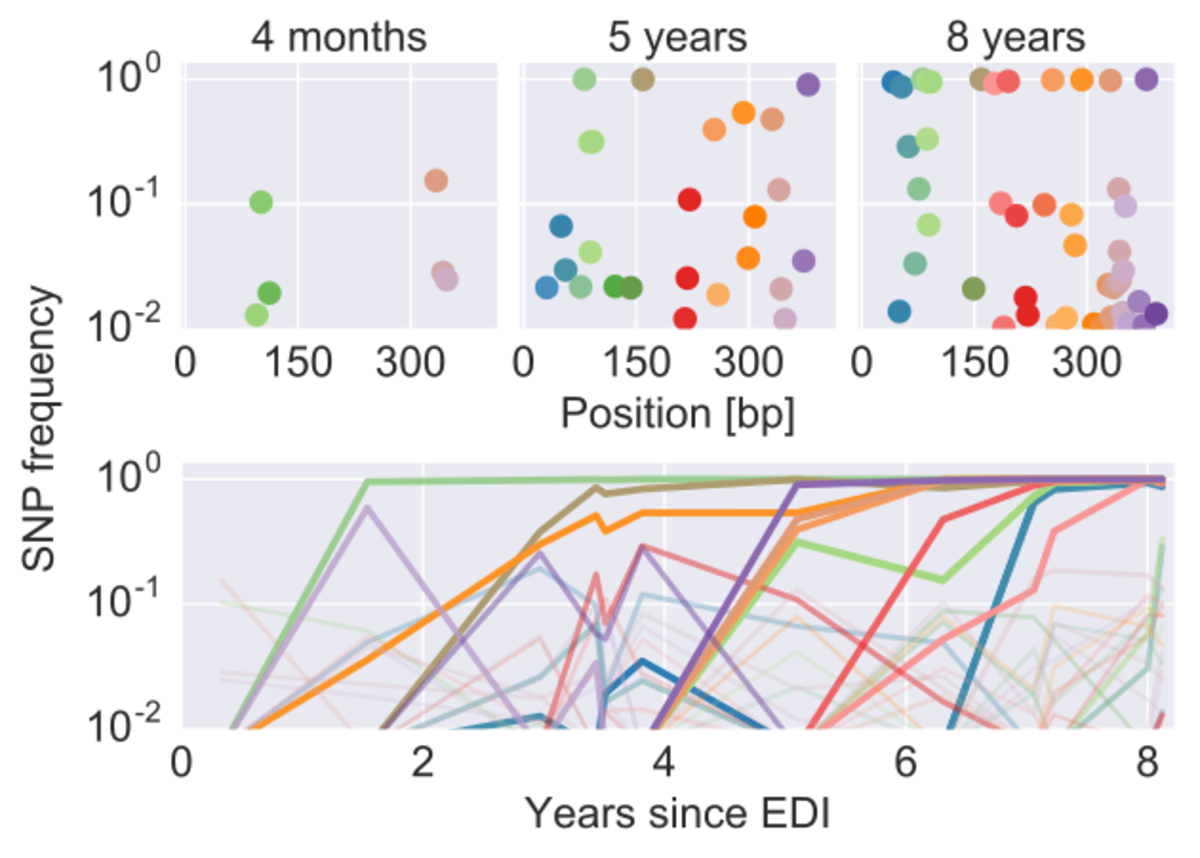
Deep sequencing of longitudinal samples of HIV populations reveals the detailed evolutionary dynamics of the virus. Each dot corresponds in the upper panels corresponds to a single nucleotide variant in the p17 gene of HIV at different time points. The lower panel shows the trajectories of these mutations through time.
Antibiotic resistance evolution
Pathogenic bacteria are increasingly resistant against many antibiotics we use to treat infections and resistance is developing into a serious complication of modern medicine. Matthias Willmann and Silke Peter from the Tübingen medical school and our group teamed up to study evolution of colistin resistance in clinical isolates of Pseudomonas aeruginosa using a morbidostat. This computer controlled continuous culture device was designed by Erdal Toprak and colleagues in Roy Kishony's lab. The morbidostat adjust drug concentrations such that the bugs struggle but grow. Over days and weeks, the bacteria become resistant while we can take samples and sequence the population to track the changes in their genomes. Over 2-3 weeks, all replicate cultures became resistant via mutational pathways that are reproducible, but strain dependent ( Regenbogen et al. AAC, 2017).
Bacterial pan-genomes and genome dynamics
In addition to vertical inheritance, bacteria exchange plasmids and genomic DNA horizontally. This mix of inheritance patterns make the analysis of bacterial evolution challenging and interesting. To come to grips the complexity of bacterial genomes, Wei Ding has developed a pipe line to reconstruct and visualize bacterial pan-genomes (Ding et al. 2017). The output of this tool is available for exploration at pangenome.ch. We are currently exploring different ways to construct, visualize, and comprehend the evolutionary laws governing bacterial diversity.
Rapid inference of time stamped phylogenies
Mutations that accumulate in the genome of replicating biological organisms can be used to infer their evolutionary history. In case of measurably evolving organisms genomes often reveal their detailed spatio-temporal spread. Such phylodynamic analyses are particularly useful to understand the epidemiology of rapidly evolving viral pathogens. The volume of genome sequences available for different pathogens, however, have increased dramatically over the last couple of years and traditional methods for phylodynamic analysis scale poorly with growing data sets. Pavel Sagulenko developed TreeTime, a python based framework for phylodynamic analysis using an approximate Maximum Likelihood approach. TreeTime can estimate ancestral states, infer evolution models, reroot trees to maximize temporal signals, estimate molecular clock phylogenies and population size histories. The run time of TreeTime scales linearly with data set size. TreeTime is available as open source package on github and as a web server at treetime.ch.