Main Content
Context-aware computational models of molecular interactions
We bring biochemical context to deep learning models for studying variability in structures and interactions of proteins.
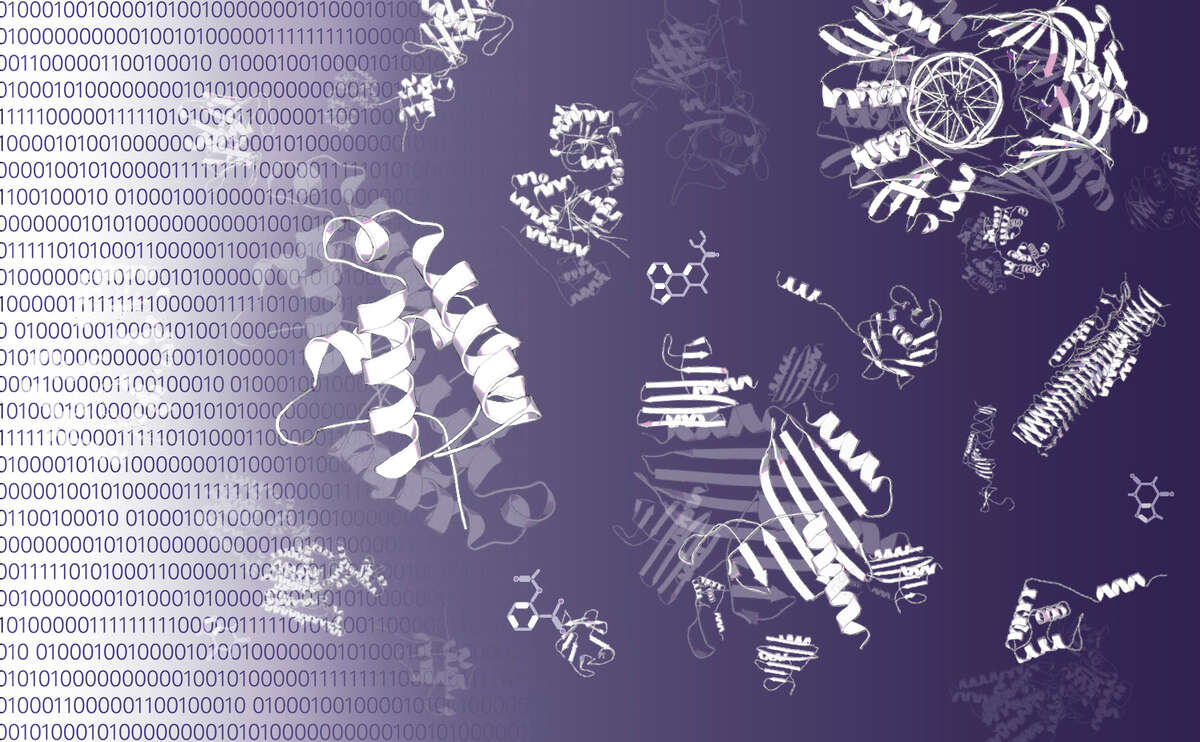
Integrating vast amounts of information about proteins into deep learning models to enable accurate prediction of protein behavior.
Interactions of proteins with molecules in their biochemical environment give rise to their biological function. These interactions and their variation are key to understanding how proteins work and designing effective therapeutics that target them.
Deep learning provides a basis for representing protein biology using the vast amounts of protein sequences and structures available. Building on this foundation allows probing protein variation through profiling of interactions and 3D structure modelling.
Adding context to protein complex prediction
We expand protein deep learning models such as AlphaFold2 by integrating data about biochemical environments. This includes information about other proteins, small molecules, sequence variations, conformations, and other experimental data to predict protein complexes at a level that allows interpretation of interactions at atomic scale.
Finding hidden connections across the protein universe
We adapt learned representations from protein deep learning models to capture remote functional associations between proteins. Specifically, we aim to identify proteins with matching binding patterns, despite low global similarity.
Characterising conserved binding interfaces and immunogenic regions
Protein structure and function prediction is complicated in scenarios with high sequence and structure variability. This includes viral proteins, immune interactions, and other specialised binding modes. We use our methods to characterise protein binding and to enable discovery of new therapeutics.
Team
Staff member
Peter Škrinjar Biozentrum, Room: 08.Q2