Main Content
Computational structure biology
Protein structure modeling
The main interest of our group is the development of methods and algorithms for molecular modeling and simulations of three-dimensional protein structures and their interactions. One of the major limitations for using structure-based methods in biomedical research is the limited availability of experimentally determined protein structures. Prediction of the 3D structure of a protein from its amino acid sequence remains a fundamental scientific problem, and it is considered as one of the grand challenges in computational biology. Comparative or homology modeling, which uses experimentally elucidated structures of related protein family members as templates, is currently the most accurate and reliable approach to model the structure of the protein of interest. Template-based protein modeling techniques exploit the evolutionary relationship between a target protein and templates with known experimental structures, based on the observation that evolutionarily related sequences generally have similar 3D structures.
The SWISS-MODEL expert system developed by our group is a fully automated web-based workbench, which greatly facilitates the process of computing of protein structure homology models. With more than 1’500 citing articles each year and about 3 models calculated per minute, SWISS-MODEL is one of the most widely used modelling servers world-wide.
Model quality estimation
Ultimately, the quality of a model determines its usefulness for different biomedical applications such as planning mutagenesis experiments for functional analyses or studying protein-ligand interactions, e.g. in structure based drug design. The estimation of the expected quality of a predicted structural model is therefore crucial in structure prediction. Especially when the sequence identity between target and template is low, individual models may contain considerable errors. To identify such inaccuracies, scoring functions have been developed which analyze different structural features of the protein models in order to derive a quality estimate. To this end, we have introduced the composite scoring function QMEAN, which consists of four statistical potential terms and two components describing the agreement between predicted and observed secondary structure and solvent accessibility. QMEANBrane further extends the approach to membrane protein structures, which play crucial roles in many biological processes and are important drug targets. Recently, we have developed an approach for dynamically combining the knowledge-based statistical potentials of QMEAN with distance constraints derived from homologous template structures (QMEANDisCo). This method significantly increases the accuracy of the local per-residue quality estimates at a relatively small computational cost, as demonstrated by the results of the community wide CAMEO and CASP XIII experiments.
CASP and CAMEO: Critical assessment of structure prediction methods
Methods for structure modeling and prediction have made substantial progress over the last decades, but still fall short in accuracy compared to high-resolution experimental structures. Assessing the quality of a blind prediction in comparison to experimental reference structures allows benchmarking the state-of-the-art in structure prediction and identifying areas which need further development. The Critical Assessment of Structure Prediction (CASP) experiment has for the last 25 years assessed the progress in the field of protein structure modeling. To this end, every two years, about 100 blind prediction targets are carefully evaluated by human experts. The “Continuous Automated Model EvaluatiOn” (CAMEO) project aims to complement CASP and provide a fully automated blind assessment for prediction servers only based on weekly pre-released sequences of the Protein Data Bank PDB. CAMEO requires the development of novel scoring methods such as lDDT, which are robust against domain movements to allow for automated continuous operation without human intervention. CAMEO is currently assessing predictions of 3-dimensional structures, residue-residue contact prediction, and model quality estimation.
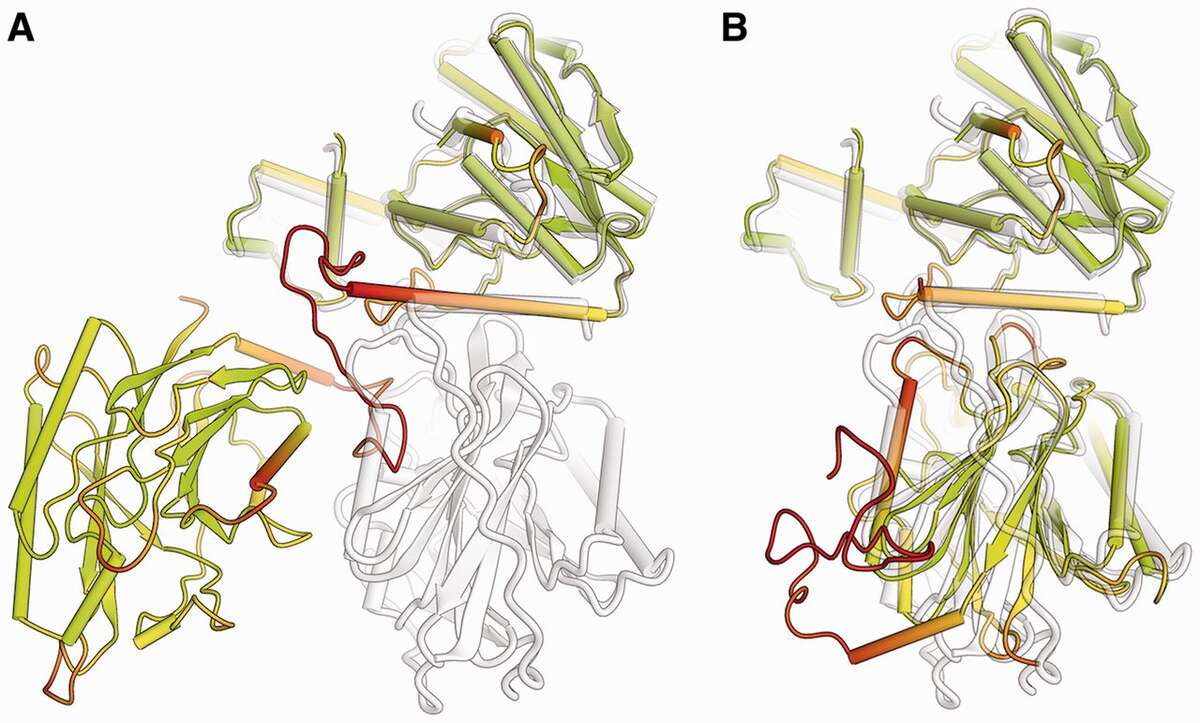
Comparison of a predicted two-domain protein structure model (colored according lDDT score) with its reference structure (shown in gray). The model is shown in full length (A), with the first domain superposed to the target. For graphical illustration, (B) shows the two domains in the prediction separated and superposed individually to the target structure. (Bioinformatics, 2013, 29:2722-2728).
Variant interpretation: From protein engineering to drug resistance
Understanding the phenotypic effect of genetic variants is one of the challenges in molecular biology. Three-dimensional models of proteins are valuable tools for the interpretation of amino acid variations – for protein engineering, in-vitro evolution experiments, the analysis of disease related mutations, or variations leading to drug resistance.
In collaboration with the SwissTPH, we investigate the mechanisms of antibiotic resistance in Mycobacterium tuberculosis (TB). Experimental analysis of resistance variants in TB is hindered by slow growth rates and difficult handling in the laboratory. We aim at developing computational approaches for predicting molecular resistance mechanisms of new genomic variants using a structure-based modelling approach. Linking genomic variants to a mechanism of action, like drug binding affinity change or abrogated activation of a prodrug, will allow us to not only make statements on the probability of resistance induced by a genetic variant, but also provide hypotheses on a molecular level how this resistance is achieved. In collaboration with the TB Research Unit at SwissTPH, we will investigate how well the method can predict resistance on real-life clinical data, especially data containing resistance variants underrepresented in the literature. The ambition of this branch of research is to generalize the method into the structural resistance variant interpretation of other drug resistant bacteria, as well as other forms of drug resistance e.g. in oncology.
Cellular therapy is a powerful therapy option, but often associated with severe unwanted side effects. Transgenes and/or genetic engineering of the transferred cells can cause malignant transformation. For example the transfer of CAR-T cells can lead to severe on- and off-target effects (cytokine release syndrome) and transfer of allogeneic T cells can cause graft versus host disease (GvHD). The success of cellular therapy in oncology will likely boost cell therapies for other indications, including non-malignant diseases. To increase the safety of cellular therapies it is important to ensure that transferred cells remain safe for years after transfer. We are collaborating with the DBM in engineering cells with mutant but functional cell surface proteins. This allows for the possibility to selectively deplete the transferred cells via a “safety or kill switch” in instances where severe unwanted side effects develop - which represents a significant increase in the safety of cellular therapy.